The Difficulty of Replicating Hot Dogs
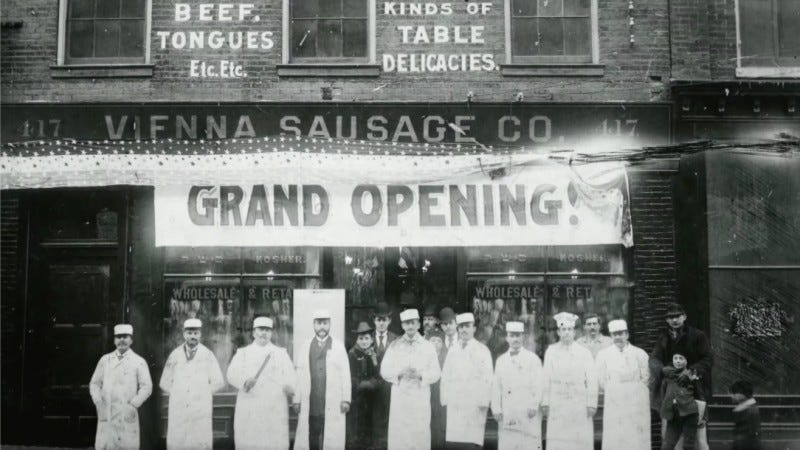
Let’s start off with a story that was featured on This American Life in 2003. It’s about the original Vienna Sausage hot dog factory in Chicago, and what happened when it moved into a new, modern building. As host Ira Glass puts it, the hot dogs just weren’t the same, and no one could figure out why:
They tasted OK, he says, but they didn’t have the right snap when you bit into them. And even worse, the color was wrong. The hot dogs were all pink instead of bright red. So they tried to figure out what was wrong.
The ingredients were all the same, the spices were all the same, the process was all the same. Maybe it was the temperature in the smokehouse. Maybe the water on the north side of Chicago wasn’t the same as the water on the South side.
They searched. They searched for a year and a half. Nothing checked out.
How complicated can it be to make a Vienna Sausage hot dog? Why would it be that hard to replicate a hot dog from one factory to the other?
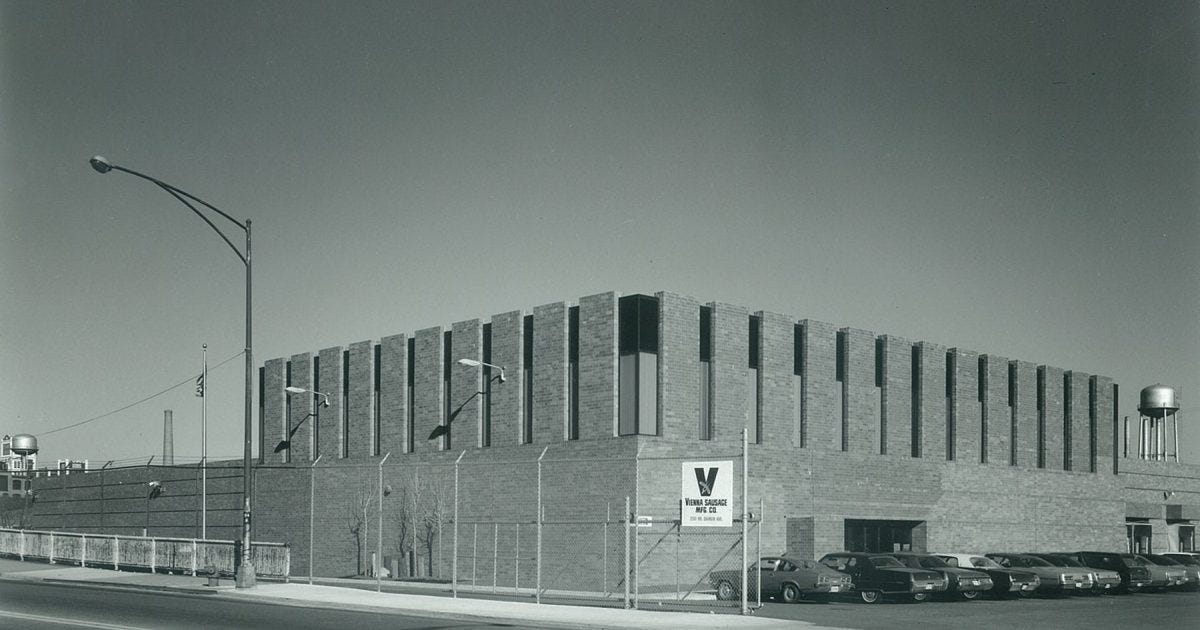
Then, some of the workers started exchanging war stories about a man named Irving, who had previously worked at the old factory. Irving’s job had been to transport the uncooked hot dogs from one part of the factory to another place where they would be cooked. In the words of Jim Bodman (president of Vienna Sausage at the time):
He [Irving] would go through the hanging vents. That’s where we hang the pastrami pieces, and it’s quite warm. And he would go through the boiler room, where we produced all the energy for the plant. He would go next to the tanks where we cook the corned beef, finally get around the corner, and in some cases, actually go up an elevator. And then he would be at the smokehouse. He would put it in the smokehouse and he would cook it.
But it turned out that in the new plant, there was “no maze of hallways,” and “no half-hour trip where the sausage would get warm before they would cook it.” In the new factory, the hot dogs were cooked right away.
It turned out that “Irving’s trip was the secret ingredient that made the dogs red. So secret, even the guys who ran the plant didn’t know about it.”
Hot dogs? Much more complicated than anyone thought.
What about scientific experiments that are more complicated than hot dogs?
The Difficulty of Replicating Cancer Biology Experiments
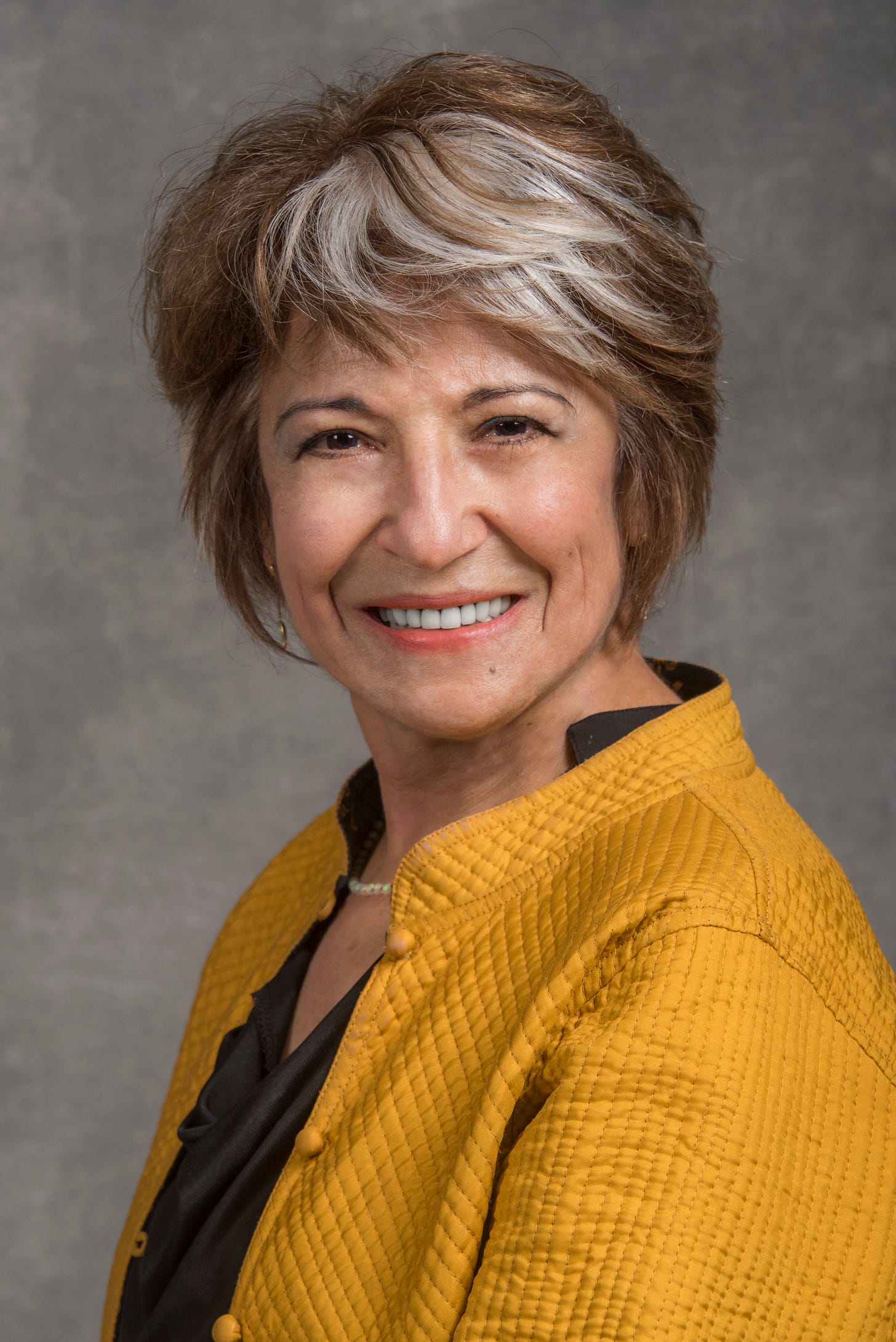
In 2014, cell biologist Mina Bissell of the Lawrence Berkeley National Lab was collaborating with scholars at the Dana-Farber Cancer Institute at Harvard on an experiment involving breast cancer cells.
They were frustrated: “Despite using seemingly identical methods, reagents, and specimens, our two laboratories quite reproducibly were unable to replicate each other’s fluorescence-activated cell sorting (FACS) profiles of primary breast cells.”
They tried everything: The instrumentation. The “specific sources of tissues…, media composition, source of serum and additives, tissue processing, and methods of staining cell populations.” The protocols to ensure they were using “identical enzymes, antibodies, and reagents.”
After doing all of this for a year, they were still stumped. So they met in person to “work side by side so we could observe every step of each other’s methods.”
In the end, they figured out that the ONLY reason for their discrepant results was this: The rate of stirring collagenase. At one lab, the tissue was stirred at a rate of 300 to 500 revolutions per minute for six to eight hours. At the other lab, it was stirred at a much more gentle rate of 80 revolutions per minute for 18 to 24 hours.
That was it. That was the one and only difference that explained why Harvard and Berkeley labs were getting such different results from an identical experiment. No one had even thought to mention the rate of stirring, because it seemed so routine and unlikely to matter.
***
Whether you’re studying breast cancer cells or making hot dogs, the task may be more complicated than you can imagine. You can get wildly different results based on factors that you never even thought of.
“Tacit knowledge” is one way of putting it, but that term seems to underestimate the problem here. To me, “tacit knowledge” describes things like my ability to ride a bicycle even though I can’t necessarily articulate and describe in words exactly how to control one’s balance.
But here, we have critically important factors that absolutely can be articulated and described . . . someday . . . maybe . . . if you spend enough time obsessively looking for them.
The problem here isn’t putting things into words. The problem is that it can take a year or more just to get the slightest idea about what’s important even when it’s been right in front of your nose the whole time.
Even more unnerving is that we have no systematic way of knowing how much this kind of thing is pervasive throughout all of science.
So what does this mean?
I see two possibilities.
The Value of Replication.
Some people are skeptical of the value of funding replications. Better to just fund original studies, and let the replications sort themselves out.
This seems wrong. Never mind the role of replication in detecting p-hacking and fraud. Instead, assume that every scientist and every publication is 100% honest. Even so, replication would be incredibly important.
After all, imagine that in the case above, the Harvard-affiliated lab did its own study on breast cancer cells, and published a certain result. Then later, the Berkeley lab with Mina Bissell decided to extend that first result by studying a different type of cell (say, based on the hormone receptors involved).
If they got a different result from the Harvard lab, they might well attribute that difference to the wrong thing — to the cells involved, rather than to the rate of stirring collagenase.
More abstractly: Imagine that there are 50 things to get “right” to see a particular result in a given experiment, and that researchers in the field are explicitly aware of only 40 of them (this may well be optimistic).
If someone tries to extend a given experiment in a new direction, they might unwittingly change 1 to 10 of the unknown but critically important factors. Any new result could be contaminated by having changed so many critically important factors at once.
Direct replication (whether simultaneous, as in a multi-site study, or asynchronous) seems like one of the best ways of figuring all of this out.
Policy conclusion #1: Agencies like NIH and NSF should fund more replication and multi-site studies, and journals should publish such studies more often.
AI-Driven Science
There’s a fair bit of hype right now about the potential for AI models to advance science by, say, scouring through millions of scientific articles and coming up with new hypotheses to test. (See here, here, here, etc.).
Some of the hype may be justified, at least someday. AI does have a lot of potential, but I suspect it’s going to be more in the realm of specialized tools (e.g., AlphaFold) trained on large, systematic datasets that are mostly accurate and complete, not LLMs trained on the published scientific literature itself.
The reason: No matter how good an LLM (or any other AI model) might be, the underlying literature just isn’t good enough. It’s often irreproducible (or even fraudulent) in ways that few people bother to check, and even when it’s relatively decent science, it is rarely (if ever) described in nearly enough detail.
As the Reproducibility Project in Cancer Biology found out, even trying to replicate studies is fraught with difficulty:
The initial aim of the project was to repeat 193 experiments from 53 high-impact papers, using an approach in which the experimental protocols and plans for data analysis had to be peer reviewed and accepted for publication before experimental work could begin. However, the various barriers and challenges we encountered while designing and conducting the experiments meant that we were only able to repeat 50 experiments from 23 papers.
Here we report these barriers and challenges. First, many original papers failed to report key descriptive and inferential statistics: the data needed to compute effect sizes and conduct power analyses was publicly accessible for just 4 of 193 experiments. Moreover, despite contacting the authors of the original papers, we were unable to obtain these data for 68% of the experiments. Second, none [!!!!!] of the 193 experiments were described in sufficient detail in the original paper to enable us to design protocols to repeat the experiments, so we had to seek clarifications from the original authors.
To go out on a limb: when an LLM is trained on the many millions of papers in the published scientific literature, no one is going to contact the original authors of every single paper to ask, “Please tell me what you actually did in this experiment, because it’s obvious on its face that half the details are missing.”
Even if anyone did that, you wouldn’t get meaningful answers the overwhelming majority of the time. And even if you did occasionally get a cooperative response, you would often end up stuck in the Mina Bissell situation, where no one knew what was going on until, after a year, they sat down side by side and walked through the entire experiment.
[PS, a nanotech professor at Rice similarly told me that he views the published literature as nothing more than advertising. In his words: “If I want to know what a lab is actually doing, I have to fly across the country and sit in their lab for a week.”]
No matter how good an AI algorithm is, it can’t perform magic. If all it has is “garbage in,” all it will produce is garbage.
Printed words alone are nowhere near enough to be the launching pad for future scientific advances.
Policy conclusion #2:
Funding agencies need to do more to incentivize:
- much more robust and complete discussions of methods, protocols, materials, etc.;
- videotaping lab work so that other people (and AI models) can directly see what occurred;
- creating many more high-quality datasets that can be the basis for future AI models, plus making them open and machine-readable (see the Open Datasets Initiative); and,
- travel—that is, create a streamlined initiative to give grants for people to spend 4 weeks a year (if they want) just watching another lab do its work, with no other purpose in mind. At a minimum, this grant program would spread ideas.